The Role of Machine Learning in Predictive Maintenance
Discover how machine learning enhances predictive maintenance, reducing downtime and costs across industries. Learn benefits, challenges, and future trends.
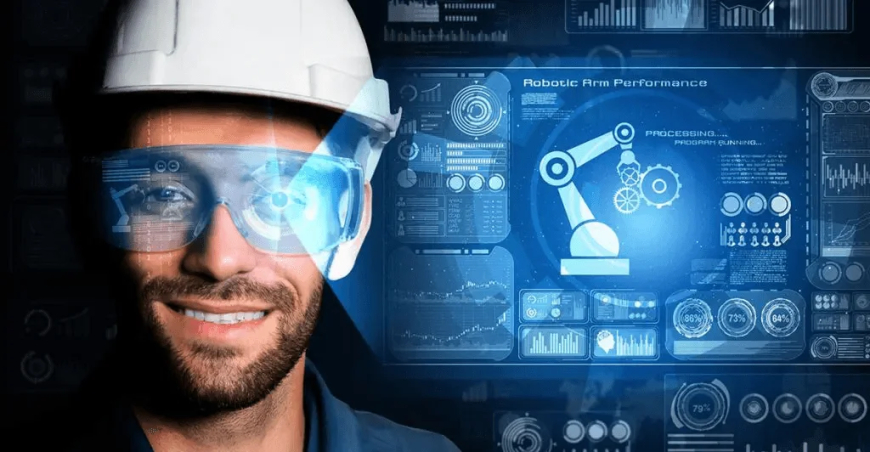
Introduction
Predictive maintenance is a game-changer in modern industries. It helps companies anticipate equipment failures before they happen, saving time, money, and resources. Machine learning (ML) has emerged as a powerful tool that enhances predictive maintenance, making it more accurate and efficient. This article explores how ML transforms predictive maintenance and its impact across various industries.
Understanding Predictive Maintenance
Definition and Basic Principles
Predictive maintenance is a proactive approach that uses data analysis to predict when equipment is likely to fail. Unlike traditional methods, it doesn't wait for failures to occur. Instead, it monitors equipment in real-time to identify potential issues early.
Comparison with Reactive and Preventive Maintenance
-
Reactive Maintenance: This is the most basic form, where repairs are made only after a failure occurs. It's costly and leads to significant downtime.
-
Preventive Maintenance: This involves regular, scheduled maintenance to prevent failures. While it's more proactive than reactive maintenance, it can still be inefficient and costly.
-
Predictive Maintenance: This uses data and analytics to predict failures before they happen, allowing for timely interventions and minimizing downtime.
Benefits
Predictive maintenance offers several benefits, including:
-
Cost Savings: By predicting failures, companies can avoid costly repairs and replacements.
-
Reduced Downtime: Early detection of issues means less downtime and more productivity.
-
Improved Safety: Predicting failures helps prevent accidents and hazardous situations.
How Machine Learning Enhances Predictive Maintenance
1. Data Collection and Analysis
Role of IoT Devices and Sensors
IoT devices and sensors are crucial for data collection. They monitor equipment in real-time, capturing data on various parameters like vibration, temperature, and pressure.
Types of Data Analyzed
The data collected includes:
-
Vibration Data: Helps detect mechanical issues.
-
Temperature Data: Indicates overheating or cooling problems.
-
Pressure Data: Monitors fluid systems for leaks or blockages.
Importance of Real-Time Data Processing
Real-time data processing is essential for timely interventions. It allows for immediate analysis and action, preventing potential failures from escalating.
2. Pattern Recognition and Anomaly Detection
ML’s Ability to Identify Patterns
Machine learning algorithms can identify patterns in equipment behavior that humans might miss. This includes subtle changes that indicate early signs of wear and tear.
Detecting Early Signs of Wear and Tear
By analyzing historical data, ML models can detect anomalies that signal impending failures. This early detection allows for proactive maintenance.
Examples of ML Models Used for Anomaly Detection
-
Support Vector Machines (SVM): Effective for classification tasks.
-
Neural Networks: Good for complex pattern recognition.
-
Random Forests: Useful for handling large datasets.
3. Predicting Equipment Failures
ML Algorithms Forecasting Potential Failures
ML algorithms like regression and classification models can forecast when equipment is likely to fail. This predictive capability is crucial for timely interventions.
Applications of Regression and Classification Models
-
Regression Models: Predict continuous values, such as the remaining useful life of equipment.
-
Classification Models: Categorize data into classes, such as "normal" or "faulty."
Benefits
-
Timely Interventions: Allows for maintenance before failures occur.
-
Resource Optimization: Ensures that resources are used efficiently.
4. Optimizing Maintenance Schedules
Dynamic Scheduling Based on ML Insights
ML insights help in creating dynamic maintenance schedules that adapt to real-time data. This ensures that maintenance is performed only when necessary.
Benefits of Reducing Unnecessary Maintenance
-
Cost Savings: Avoids unnecessary maintenance costs.
-
Improved Efficiency: Focuses resources on areas that truly need attention.
Examples of Industries Using Optimized Schedules
-
Manufacturing: Optimizes machinery maintenance.
-
Energy: Ensures reliable power supply.
-
Transportation: Keeps vehicles and fleets running smoothly.
Machine Learning Techniques in Predictive Maintenance
5. Supervised Learning
Training Models with Labeled Data
Supervised learning involves training ML models with labeled data. This data includes examples of both normal and faulty equipment behavior.
Examples of Use Cases and Algorithms
-
Use Cases: Predicting engine failures, detecting anomalies in manufacturing lines.
-
Algorithms: Linear regression, decision trees, neural networks.
6. Unsupervised Learning
Identifying Hidden Patterns Without Labeled Data
Unsupervised learning identifies patterns in data without the need for labeled examples. This is useful for discovering unknown anomalies.
Applications in Clustering and Anomaly Detection
-
Clustering: Groups similar data points together.
-
Anomaly Detection: Identifies outliers that may indicate failures.
7. Reinforcement Learning
Adaptive Learning for Complex Systems
Reinforcement learning allows ML models to learn from their environment through trial and error. This is particularly useful for complex systems where the behavior is hard to predict.
Examples of Real-World Applications
-
Robotics: Teaching robots to perform maintenance tasks.
-
Autonomous Vehicles: Optimizing routes and maintenance schedules.
Applications of Predictive Maintenance Across Industries
8. Manufacturing
Monitoring Machinery and Assembly Lines
In manufacturing, predictive maintenance monitors machinery and assembly lines to ensure smooth operation. Sensors collect data on machine performance, which ML models analyze to predict failures.
Case Studies of Predictive Maintenance in Factories
-
Automobile Industry: Predicting failures in assembly lines.
-
Food Processing: Ensuring machinery runs smoothly to avoid production halts.
9. Energy and Utilities
Predicting Failures in Power Grids and Turbines
In the energy sector, predictive maintenance is crucial for predicting failures in power grids and turbines. This ensures a reliable supply of energy.
Role in Optimizing Renewable Energy Systems
ML models help optimize renewable energy systems by predicting maintenance needs for solar panels, wind turbines, and other renewable sources.
10. Transportation and Logistics
Monitoring Vehicle Performance and Fleet Management
In transportation, predictive maintenance monitors vehicle performance and helps in fleet management. Sensors on vehicles collect data on engine performance, tire pressure, and more.
Examples from Aviation, Shipping, and Railways
-
Aviation: Predicting engine failures and optimizing maintenance schedules.
-
Shipping: Ensuring vessels are seaworthy and reducing downtime.
-
Railways: Monitoring train performance and predicting track issues.
11. Healthcare and Medical Equipment
Ensuring Reliability of Critical Medical Devices
In healthcare, predictive maintenance ensures the reliability of critical medical devices. This is crucial for patient safety and effective treatment.
Applications in Hospitals and Diagnostic Centers
-
Hospitals: Monitoring equipment like MRI machines and ventilators.
-
Diagnostic Centers: Ensuring accuracy and reliability of diagnostic tools.
12. Oil and Gas Industry
Monitoring Pipelines and Drilling Equipment
In the oil and gas industry, predictive maintenance monitors pipelines and drilling equipment to prevent costly downtimes and environmental disasters.
Predicting and Preventing Costly Downtimes
ML models analyze data from sensors on pipelines and drilling equipment to predict and prevent failures, ensuring continuous operation.
Benefits of ML-Driven Predictive Maintenance
13. Cost Efficiency
Reducing Repair and Replacement Expenses
ML-driven predictive maintenance reduces repair and replacement expenses by predicting failures before they occur. This allows for timely and cost-effective interventions.
Extending Equipment Lifespan
By predicting and addressing issues early, predictive maintenance extends the lifespan of equipment, saving on replacement costs.
14. Enhanced Safety
Preventing Accidents and Hazardous Situations
Predictive maintenance enhances safety by preventing accidents and hazardous situations. Early detection of issues helps avoid catastrophic failures.
Role in High-Risk Industries
In high-risk industries like oil and gas, healthcare, and transportation, predictive maintenance is crucial for ensuring safety and preventing disasters.
15. Improved Productivity
Minimizing Downtime and Operational Disruptions
Predictive maintenance improves productivity by minimizing downtime and operational disruptions. This ensures that equipment runs smoothly and efficiently.
Enhancing Overall Efficiency
By optimizing maintenance schedules and reducing unnecessary maintenance, predictive maintenance enhances overall efficiency.
Challenges in Implementing ML for Predictive Maintenance
16. Data Quality and Availability
Importance of Accurate and Sufficient Data
The success of ML-driven predictive maintenance depends on the quality and availability of data. Accurate and sufficient data is essential for reliable predictions.
Addressing Challenges in Data Collection
Challenges in data collection include ensuring sensors are accurate and data is collected consistently. Addressing these challenges is crucial for effective predictive maintenance.
17. High Initial Investment
Costs of Deploying ML Systems and IoT Infrastructure
Deploying ML systems and IoT infrastructure requires a high initial investment. This includes the cost of sensors, data storage, and ML tools.
Strategies for Achieving ROI
To achieve a return on investment (ROI), companies should focus on long-term benefits like reduced downtime, cost savings, and improved efficiency.
18. Expertise and Training
Need for Skilled Professionals to Manage ML Systems
Implementing ML for predictive maintenance requires skilled professionals who can manage and interpret ML systems. This includes data scientists, ML engineers, and maintenance experts.
Importance of Upskilling and Continuous Learning
Upskilling existing staff and continuous learning are essential for keeping up with the latest ML technologies and best practices.
Future Trends in ML for Predictive Maintenance
19. Integration with AI and IoT
Combining AI, IoT, and ML for Smarter Systems
The future of predictive maintenance lies in the integration of AI, IoT, and ML. This combination creates smarter systems that can predict failures with even greater accuracy.
Examples of Emerging Technologies
-
AI-Driven Analytics: Provides deeper insights into equipment behavior.
-
Advanced IoT Sensors: Collect more detailed and accurate data.
20. Cloud-Based Predictive Maintenance
Role of Cloud Computing in Data Storage and Processing
Cloud computing plays a crucial role in data storage and processing for predictive maintenance. It offers scalability and accessibility, making it easier to manage large datasets.
Benefits of Scalability and Accessibility
Cloud-based solutions provide scalability, allowing companies to handle increasing amounts of data. They also offer accessibility, making it easy to access data and insights from anywhere.
21. Edge Computing and Real-Time Analysis
Performing ML Computations Closer to the Data Source
Edge computing performs ML computations closer to the data source, reducing latency and improving real-time decision-making.
Advantages for Real-Time Decision-Making
Edge computing offers advantages for real-time decision-making, allowing for immediate interventions and preventing failures before they occur.
Tips for Businesses Adopting ML for Predictive Maintenance
Assessing Equipment and Data Readiness
Before adopting ML for predictive maintenance, businesses should assess their equipment and data readiness. This includes ensuring that equipment is compatible with sensors and that data collection systems are in place.
Choosing the Right ML Tools and Platforms
Choosing the right ML tools and platforms is crucial for effective predictive maintenance. Businesses should consider their specific needs and the capabilities of different ML tools.
Collaborating with Experts and Vendors
Collaborating with experts and vendors can provide valuable insights and support. This includes working with data scientists, ML engineers, and vendors who specialize in predictive maintenance solutions.
Conclusion
Machine learning has a transformative role in predictive maintenance, offering numerous benefits across various industries. By adopting ML-driven predictive maintenance, businesses can achieve long-term benefits like cost savings, enhanced safety, and improved productivity. The future of maintenance strategies lies in the integration of ML, AI, and IoT, creating smarter and more efficient systems.
Freqyently Asked Questions (FAQs)
1. What is predictive maintenance and why is it important?
Predictive maintenance is a proactive approach that uses data analysis to predict equipment failures before they happen. It's important because it helps reduce downtime, save costs, and improve safety.
2. How does machine learning enhance predictive maintenance?
Machine learning enhances predictive maintenance by analyzing data to identify patterns and predict failures. This allows for timely interventions and optimized maintenance schedules.
3. What industries benefit the most from ML-driven maintenance?
Industries that benefit the most from ML-driven maintenance include manufacturing, energy, transportation, healthcare, and oil and gas. These industries rely heavily on equipment and can gain significant advantages from predictive maintenance.
4. What are the challenges of implementing ML for maintenance?
Challenges include data quality and availability, high initial investment, and the need for skilled professionals. Addressing these challenges is crucial for successful implementation.
5. What are the future trends in predictive maintenance?
Future trends include the integration of AI and IoT with ML, cloud-based solutions, and edge computing for real-time analysis. These trends aim to create smarter and more efficient maintenance systems.
What's Your Reaction?
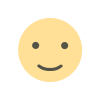
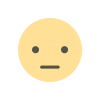
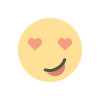
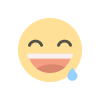

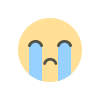
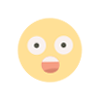