Understanding Artificial Intelligence (AI) and Machine Learning (ML)
Discover the differences between AI and ML, their applications, benefits, and how they transform industries through data-driven insights and automation.
Introduction
AI and Machine Learning are among the major technologies laying a foundation for our existing world. Even though they have usually been spoken of in one breath, they are different concepts, playing different roles in data handling, decision-making, and automation.
Artificial Intelligence and Machine Learning: What is this?
Artificial Intelligence is the general area that includes all sorts of technologies intended to make machines perform the same way as human cognitive skills: reasoning, learning, problem-solving, perception, and language understanding. AI systems analyze data, provide recommendations, and perform tasks that typically require human intelligence.
While AI is a general field that concerns intelligent machines, Machine Learning narrows its scope to algorithms which provide the ability for machines to learn from data. Rather than explicit programming for performance, the ML system looks for patterns in data and automatically gets better with more exposures.
AI and ML: Relationship
The relationship of AI to ML can be viewed as AI being the general term, embracing a number of approaches and technologies, with ML being one of its major milestones. Other sub-branches working under AI include deep learning, NLP, and robotics.
For instance, if you ask your voice assistant Siri or Google Assistant how long it takes to commute somewhere, it is AI that tells the system what you want and provides an answer. The ML algorithms hidden in the background use historic data on traffic flow to estimate travel times according to any particular pattern.
Key Differences between AI and ML
Scope:
AI applies to robotics, natural language processing, and complex problem-solving tasks involving healthcare, finance, among other major industries. The scope goes way beyond just creating algorithms for predictions and classification issues. Functionalities:
AI aims at building systems that are able to perform tasks intelligently. It covers both rule-based learning and data-driven learning.
ML involves an algorithm learning from data such that its performance gets better with time without interference from humans while it is being trained. Application:
General applications of AI include unmanned vehicles, digital assistants, face detection, among others.
On the other hand, this domain applied in recommendation systems like Netflix, the fraud detecting system in banks, and Predictive Analytics in the Health Sector.
Business Benefits AI and ML
Business today uses AI as well as ML to improve processes for efficiency and enable good decision-making. Some of the key benefits of these include:
- Greater Use of Data: With the incorporation of both, organizations can analyze structured and unstructured data.
Speedier Decision Making: Automation of data processing will lead to quicker and better decisions with less human error for the companies. - Operational Efficiency: The routine tasks that get automated will lead to greater productivity and lesser costs.
- Smarter Analytics: The integration of predictive analytics within business processes will have employees making better decisions based upon data.
Applications in Real-World
AI and ML are causing a revolutionary turn in industries, enabling innovative solutions:
Healthcare-to gain insight from patient health records, forecast outcomes, accelerate drug development, enhance diagnostics. Manufacturing-optimize production machines for predictive maintenance and improvement of operational efficiency with IoT analytics. Retail-inventory management and demand forecasting, offering a personalized shopping experience with recommendation engines. Financial Risk Assessment: it detects fraud in real time, automation in trading processes, optimization of service delivery.
Challenges and Considerations
Amidst all the good things that come out of AI and ML, there is a number of challenges too:
- Data Quality: Success for any algorithm in ML is quite heavily dependent on input quality. Poor-quality data can lead to less-than-accurate predictions.
- Ethical Issues: The concept of AI has ethical issues related to privacy, surveillance, biased decision algorithms, and job losses due to automation.
- Complexity in Implementation: AI and ML can be cumbersome to implement because most companies need to integrate them into their running business processes. Skilled manpower will also be required to accomplish this task successfully.
Conclusion
Artificial Intelligence and Machine Learning are not just buzzwords but powerful tools for massive leaps forward across industries. The differentiation between and interrelation of the two needs to be well understood by an organization intending to use them to its full potential. As these technologies continue to evolve, so will their applications expand, making smarter decisions and operations possible in a world increasingly driven by data. Embracing such technologies will be part of the future landscape if businesses are to remain competitive.
What's Your Reaction?
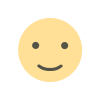
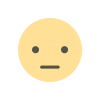
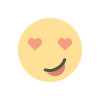
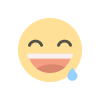

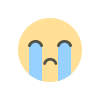
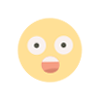